The company now has $15m in funding not including the conversion of $2.5m in initial seed money. In February 2017, it signed a partnership agreement with Yokogawa Electric (one the original investors) to develop and promote the use of 'fog computing' products for industrial IoT (IIoT). The company has now extended the capabilities of its software to include machine-learning capabilities with Lightning ML, running at the edge in a very low-memory footprint for OT devices.
FogHorn has evolved quickly with the IIoT industry. Last year, it promoted the importance of edge analytics in OT. Because the edge is becoming a normal part of any IoT conversation and a path to pursue for many vendors, FogHorn is pushing on with machine learning (ML). Such capabilities are generally assumed to be high-end supercomputer and cloud functions. In specifically using ML, the company helps further shift the mindset of its industrial customers from assuming cloud-first for IIoT. Injecting its codebase into lower-level devices in industrial facilities, and tuning its development tools for that audience, allows the company to start setting the standards for massively distributed processing – the 'fog' in FogHorn. Working on the US West Coast, but also pulling in industrial giants, has put the company at the major cultural intersection of IT and OT. Practical deployments show its effectiveness in solving problems. FogHorn will face competition from the spectrum of artificial intelligence and analytics providers, but it's gotten in early and deep.
Context
FogHorn has attracted the attention of both OT and IT companies because it represents the crossover point between the engineering requirements of industrial devices that can benefit from local real-time analysis, and the drive of the IT industry toward industrial processes with IoT. The brownfield approach to inject new functions into existing industrial systems is a major pattern for IIoT adoption.
This type of industry crossover was a key focus of our report: Industrial Internet of Things: State of Play. The initial release of Lightning was focused on edge analytics, complex event processing (CEP) for static rule sets. These rule sets could be adjusted and redeployed as tuning data surfaced.
The new software-product release has introduced the ability to run ML locally. This allows live streams of data to be analyzed at the source, and to adjust the responses in real time to control machinery. The company has introduced an EdgeML component that can import existing ML models, or run user-created ones. All this can run in a very restricted device, often less than 150MB of memory using its component architecture supporting its CEP.
The software is deployed in a Docker container, and while designed to run in the equivalent of half a Raspberry Pi, it can also be run anywhere from endpoints to the cloud. To help create and deploy EdgeML, FogHorn has added additional tooling, testing and device support.
Products
Lightning is delivered in two editions as software stacks. The Micro edition runs under 256MB, and includes EdgeML, data visualization, pre-processing and enrichment, high-speed streaming analytics and a C++ SDK for custom builds. Standard edition has all of the Micro edition features, but runs in under 2GB, adds local data storage, publication to cloud and historians, a data simulator, authoring tool and Polyglot SDK for customer development.
The company has addressed some of our previous concerns about the need to learn a new authoring language by providing OT-centric graphical authoring tool VVM, live streaming-data visualizer VIZ, and an enhanced simulator tool for multiple protocols called REX. In addition to testing with protocols such as MQTT and OPC UA, it now publishes directly back to other OT system using those protocols for tighter integration. OSIsoft PI Read/Write is used to push or pull from Pi Historians.
Lightning can now be run on ARM32 processors in addition to its previous x86 target, to further extend its IIoT potential, running on anything from PLCs. Orchestration and deployment is managed on-premises via a private datacenter. The company is enabling a common AppStore for partner applications built for ML, monitoring, predictive maintenance and operation intelligence using its Polyglot SDK.
Customers
FogHorn's customer examples included one that involved a high-value, three-stage manufacturing process where the complex end product was failing too often. Lightning was used in each of the stages of production, from the simpler end-device configuration to the initial highly complex and tuned machine that started the process off.
It had been assumed failures were occurring in the last process that involved more basic technology, with only about 30 parameters. However, it was found that the start of the process, with complex high-end machines of around 300 sensors and parameters, was the one that benefited the most from the analytics, ML and subsequent tuning to reduce the failure rates.
Competition
Edge processing is the direction many companies are taking now. Amazon's Greengrass appliance brings the datacenter to the shop floor. Microsoft Azure and IBM's Watson are both pushing function analytics and learning to the edge as well.
Products from companies such as ClearBlade run as in a full-function container at the edge. Bsquare DataV collates and wrangles data from multiple industrial sources for ingestion into any third-party analytics. We covered the complexity of data in IoT in our recent report Data Management of Things 2017.
Strengths
FogHorn has maintained and enhanced its strong support from OT and IT, and has shown continued development in bringing small-footprint ML to its portfolio.
Weaknesses
With more graphical interfaces to build elements of the system and more testing tools, it will need increased validation, checks and balances, and explicit version control as systems get more complex.
Opportunities
By approaching IIoT from the OT device side, but in a way that fits large computing resources, the company has the potential to drive truly distributed computing across the continuum.
Threats
The fast-moving evolution of IIoT from cloud to edge, and eventually to fully distributed computing, will bring plenty of competition from mega-scale IT companies.
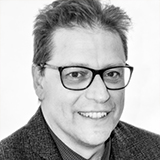
Ian Hughes is an analyst for the Internet of Things practice at 451 Research. He has more than 27 years of experience in emerging technology as a developer, architect and consultant through key technology trends.
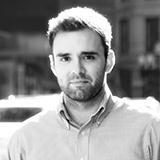
As a Senior Research Associate in 451 Research’s Information Security Channel, Patrick Daly covers emerging technologies in Internet of Things (IoT) security. His research focuses on different industrial disciplines of IoT security, including the protection of critical infrastructure, transportation and medical devices. In addition, Patrick’s coverage spans technological domains, including security for IoT devices, applications, platforms and networks.
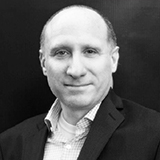
Keith Dawson is a principal analyst in 451 Research's Customer Experience & Commerce practice, primarily covering marketing technology. Keith has been covering the intersection of communications and enterprise software for 25 years, mainly looking at how to influence and optimize the customer experience.