Published: May 4, 2020
Introduction
We track use cases for AI and machine learning across multiple industries as part of our Voice of the Enterprise AI & Machine Learning survey-based research. Our most recent survey was performed in November 2019, right on the cusp of the COVID-19 outbreak. So while we didn't ask respondents how COVID-19 would affect their use of AI, we can make informed projections about the leading and lagging use cases.
In this report, we look at three industries: healthcare, financial services and retail. We will have an additional report looking at use cases in manufacturing, energy and media/entertainment.
The 451 Take
Healthcare
In 2015, the UK's NHS estimated that the annual cost of paper storage for each NHS hospital trust is between £500,000 ($622,000) and £1m. And that's before you factor in the inefficiency cost. COVID-19 is short-circuiting barriers to adoption in areas such as telemedicine, which is being adopted on a highly accelerated scale during the pandemic, mainly due to lack of alternatives.

Treatment development already showed the largest percentage point increase among this list of use cases – up 26 points in two years' time. This demonstrates that healthcare providers were already excited about expediting and scaling epidemiological and genetics studies to generate novel treatment methodologies – something that is being accelerated in ways we didn't think were even possible pre-COVID-19.
Cutting down the lengthy, difficult and expensive process of drug discovery has long been a strong use case for AI, as it involves – among many other things – trawling through vast collections of disparate information to identify patterns. The clinical trial-matching process is another area ripe for efficiency improvements using NLP to trawl through doctors' notes and other free-text that form medical data, rather than just relying on structured data in electronic medical records systems. This helps identify patients more quickly, and thus expedites the clinical trial process.
Financial Services
Financial services firms are obviously going to be tested as most of the world heads into recession, and in ways they haven't experienced since the 2007-2008 crisis. This time, the blame can't be laid at their door. And hopefully, the stress tests and other measures taken after that crash have put these firms in a better position this time around for what is a different type of shock.
Of the use cases from our survey, digital/data security topped the list, with 42% choosing it as a current use case and 56% for two years' hence. Fraud detection was second choice in two years' time. Respondents expect those two issues and back-end process automation to be the three use cases that are almost neck-and-neck. Fraud is often, of course, an unwelcome bedfellow to moments of crisis, and financial firms need to keep their customers and systems safe.
Back-end process automation is something banks have been adopting broadly over the past few years, mainly in the form of robotic processing automation (RPA). That technology sector would seem an obvious choice for seeing some benefit from a pandemic, since automating processes that are otherwise performed by humans would be a logical area for cost savings and would enable social distancing.
Payment processing was another popular machine-learning use case in our pre-COVID-19 survey that we expected to increase in popularity. As payments move to contactless by default to help maintain social distancing, fraud detection is an essential component of such an expansion to guard against false positives, especially as maximum transaction values are increased (the UK went from £30 to £45 per transaction on April 1, for example).
Another payment processing use case is revenue optimization – the routing of a transaction to a payment processor that has the highest likelihood to approve the transaction based on a variety of factors (type of card, geography, time of day).
Retail
The retail sector will undergo perhaps the most profound changes of any of the industries we are looking at in these reports. Of course, much of this is accelerating what was already happening, as consumers move to online commerce, but given that only 11.4% of US retail sales were online (according to the Census Bureau of the US Department of Commerce's Q4 2019 figure published on February 19, thus before the pandemic made an impact in the US), a huge amount of the $1.39bn US retail sales in Q4 2019 were in-store. That will now change, and we have already seen massive furloughs and layoffs from major names such as JC Penney and Macy's.
Online retail is becoming a way of life for people who previously dabbled or avoided it altogether, and the pandemic has shown that people will give online commerce a try for items they would have previously insisted going to store to buy. According to 451 Research's Voice of the Customer: Macroeconomic Outlook, Consumer Spending April 2020, more than eight in 10 people say the crisis has shifted at least some of their spending from stores to online channels. Some of that change will stick while other parts may revert.
For those retailers that survive – and many will not – a shift to a much greater percentage of sales online will change the response for number one use case for machine learning in two years' time, which was customer engagement. Our survey showed 56% of respondents expecting it to rise to the top in two years, and that timeframe is likely to be compressed by COVID-19.
If a much larger percentage of sales are online, one upside is that retailers should have more – and more accurate – data to build models with than they could have gleaned from physical stores. We've seen the effect of a coronavirus outbreak on online commerce before – in the now-famous story of how Alibaba thrived during the occurance of SARS in China in 2003, effectively launching China's online commerce industry.
If social distancing is maintained for many years, then cashier-less store rollout will accelerate. So Amazon Go may move from being an experiment to mainstream – and Amazon will have plenty of access to low-cost vacant retail space to expand Go. Physical security using AI-driven image recognition will see a large expansion as a result.
Payment processing features prominently as a retail use case, just as it does in financial services. The 'contactless by default' effect has already been observed, with MasterCard reporting 40% growth in contactless transaction volume in Q1 2020 compared to the year-ago quarter. Cash will become truly exceptional in many retail sectors, and may not be accepted at all in some places.
Supply and demand predictions had been the largest growth area predicted by our survey respondents (27 percentage points). We expected to see an even more pronounced emphasis here as supply chains shorten with the drive to source closer to home – a trend that was already happening as costs in China were rising. One upside for retailers is the potential to tailor more to local tastes, all of which takes data analytics and machine learning.
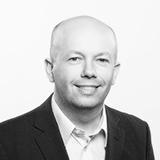
Nick Patience is 451 Research’s lead analyst for AI and machine learning, an area he has been researching since 2001. He is part of the company’s Data, AI & Analytics research channel but also works across the entire research team to uncover and understand use cases for machine learning.
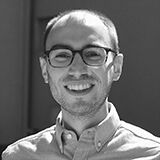
Jeremy Korn is an Associate Analyst for the Data, AI & Analytics Channel at 451 Research, where he covers artificial intelligence and machine learning in the enterprise. In particular, he focuses on the legal and ethical challenges raised by these emerging technologies. In addition, Jeremy helps lead the Voice of the Enterprise: AI and Machine Learning survey, which provides qualitative insights into AI adoption, use cases and infrastructure.
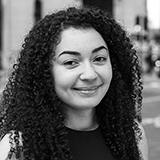
Rachel Dunning is a Research Associate at 451 Research. Prior to joining 451 Research, she graduated from Fitchburg State University Magna Cum Laude with a BS in Cognitive Psychology and Economics. While attending school, she gained exposure to research methodology and data analytics through her involvement in several academic research projects. She is conversationally fluent in German.