Introduction
The 451 Take
Primacy of cloud-based AI platforms
Cloud-based AI platforms also align with the increasingly common cloud-native approach to IT infrastructure. The technologies underpinning these products – containers, microservices, service meshes, etc. – beget the flexibility and resilience necessary for large-scale deployment of automated decision-making systems driven by machine learning application.
The Vendor Landscape
The vendor landscape in regard to cloud-based AI platforms, which has remained essentially unchanged in year-over-year data, can be divided into three tiers:
- Public cloud providers. Microsoft Azure, Amazon Web Services, IBM and Google Cloud Platform are leading the AI platform market. These providers have recognized the importance of AI capabilities both in terms of driving customers to their platforms and encouraging them to increase their usage. In addition to building out capabilities within core AI functions like computer vision and natural language processing, these companies have recently expanded features around machine learning operationalization and even moved into horizontal applications such as contact center software.
- Software vendors. Companies like Oracle, Adobe, SAP and Salesforce have been offering enterprises specialized software products for decades, and they already have vast volumes of enterprise data in these systems. From their perspective, it is important not only to infuse machine learning into their applications, but also allow customers to train and deploy their own applications using their own data. To this end, like the public cloud providers, these vendors have built out machine learning capabilities on top of their platforms.
- Pure-play vendors. There are several providers in this market – such as DataRobot, H2O.ai and C3.ai – whose primary products are cloud-based AI platforms. While these companies are relatively new to the fold, they often differentiate themselves through advanced functionality and open architecture. In some cases, they offer vertical-specific applications to help customers get up and running in niche use cases.
Factors Driving Vendor Selection
It is important to point out that this is not a winner-takes-all market because the average adopter of cloud-based AI platforms leverages multiple vendors. At this early stage of adoption, there are enough non-overlapping capabilities and distinct functionalities to justify splurging on the services of more than one vendor.
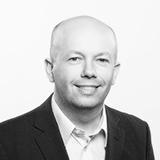
Nick Patience is 451 Research’s lead analyst for AI and machine learning, an area he has been researching since 2001. He is part of the company’s Data, AI & Analytics research channel but also works across the entire research team to uncover and understand use cases for machine learning.
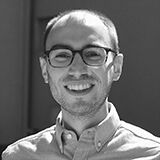
Jeremy Korn is an Associate Analyst for the Data, AI & Analytics Channel at 451 Research, where he covers artificial intelligence and machine learning in the enterprise. In particular, he focuses on the legal and ethical challenges raised by these emerging technologies. In addition, Jeremy helps lead the Voice of the Enterprise: AI and Machine Learning survey, which provides qualitative insights into AI adoption, use cases and infrastructure.
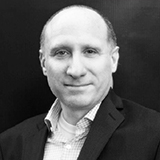
Keith Dawson is a principal analyst in 451 Research's Customer Experience & Commerce practice, primarily covering marketing technology. Keith has been covering the intersection of communications and enterprise software for 25 years, mainly looking at how to influence and optimize the customer experience.