Published: September 3, 2020
Introduction
However, the changes that impacted travel and work conditions, as well as trading and supply chains, were so significant and so sudden that the COVID-19 pandemic has also highlighted that there can be significant limitations to relying on historical data and models, as well as traditional analytics approaches that require data analysts to create dashboards and reports for decision-makers, with inherent delays to decision-making. One potential answer to these challenges is proactive intelligence – a more automated approach to enabling senior and line-of-business decision-makers to generate actionable insight from the continuous analysis of data generated by operational applications, in addition to data warehouses, data lakes and other systems of record.
The 451 Take
The ability of business leaders to quickly use data from operational applications to make strategic decisions and deliver on strategic outcomes will rapidly be seen not just as a potential competitive differentiator, but also as a fundamental requirement and strategic imperative. As such, we believe there is a bright future for proactive intelligence, including both the new breed of intelligent enterprise applications that leverage embedded analytics and AI/ML functionality to deliver departmentally specific insight, and the automated decision intelligence products that are similarly designed to automatically assess a company's entire data estate, to generate insight and recommendations delivered directly to senior decision-makers.
Shining a Light on the Strengths and Limitations of Analytics
The faster data can be leveraged by business decision-makers to make strategic decisions and take action, the more agile a company can be in improving operational efficiency, driving transformational change, and responding to changing market conditions and competitive threats.
Work practices and applications are evolving. Having a cohesive approach to analyzing, making decisions and taking action based on data is a growing requirement for both senior executive and line-of-business decision-makers. For example, 45% of respondents to 451 Research's Voice of the Enterprise: Workforce Productivity & Collaboration survey say they have seen data literacy – the ability to read, analyze, understand and communicate with data – as an increasingly important requirement for HR professionals over the last three years.
However, the pandemic has also shone a light on the limitations of current approaches to analytics to serve decision-makers. For example, we previously highlighted the importance of scenario analysis, given the high levels of uncertainty and the risks of relying on historical models and data that may no longer be relevant.
In addition to being reliant on historical data sources, data-driven decision-making has traditionally been a multi-participant process that requires the creation of business intelligence dashboards and reports, and is dependent upon collaboration between IT professionals, data analysts and business decision-makers.
This multistep process can cause delays in data access for line-of-business decision-makers in finance, marketing, logistics and human resources. Ultimately, these challenges can lead to friction between data consumers (those tasked with making data-driven decisions) and data operators (those tasked with configuring and managing data pipelines), just as they are being asked to accelerate data-driven projects.
When there is friction and delay in accessing data, it can negatively impact organizational efficiency and productivity. There is also a danger that, when faced with an abundance of different data sources and a variety of complex and time-consuming reports and dashboards, decision-makers can become overwhelmed and revert to gut feel or manual data selection approaches that are inherently biased.
These ongoing challenges are exacerbated by the current economic climate and the uncertainty related to the nature of COVID-19 quarantine measures. In particular, changes in customer, employee and supplier behavior are rendering some existing analytics and machine learning models obsolete.
Some evidence of that comes from 451 Research's recent Voice of the Enterprise: AI & Machine Learning, Infrastructure survey, in which 58% of respondents indicated that they have decelerated or halted existing AI initiatives as a direct result of the pandemic. This is not due to a lack of confidence in AI/ML overall; however, three quarters of respondents agree that COVID-19 is causing them to invest in new AI/ML initiatives.
While these two responses appear contradictory at first glance, one logical explanation is that existing models based on historical data and assumptions may no longer be fit for purpose and are being retired in favor of new initiatives. This is a natural consequence of the fact that predictive models can be impacted by model accuracy drift, and the model itself decays as time elapses and circumstances change.
In addition to further investment in new predictive models, many companies are also investing in complementary approaches to enable decision-makers to generate actionable insight from operational data without the delays and friction that can be associated with traditional analytics workflows. One of these approaches is proactive intelligence.
What is Proactive Intelligence?
Generating actionable insight from operational data produced by enterprise applications is more important than ever for strategic decision-makers. From the perspective of senior decision-makers, traditional business intelligence workflows are passive in response to changing business environments. The problem is that the terms of any business intelligence project – the data required and the desired goals – have been defined in advance. This process results in dashboards and reports that are tailored to specific business conditions and requirements, and creates inherent delays in decision-making when it comes to responding to fast-changing requirements.
Unanticipated events force decision-makers to go looking for data, and may also require the requisitioning of data, the associated infrastructure to process the requisitioned data, and data or business analysts to initiate and operate data processing pipelines before decision-makers are able to seek the answers to any new questions they may have.
Rather than requiring decision-makers to seek out new data or commission new analytics projects as conditions evolve, proactive intelligence is designed to monitor operational data in real-time, diagnose changes in business metrics and alert decision-makers about the changes, as well as the causes and implications, while recommending appropriate actions. These proactive intelligence workflows rely on continuous analysis of operational data.
Additionally, unlike traditional business intelligence tools and workflows that require active participation of data and business analysts to create reports and dashboards for business users, proactive intelligence is designed to automate the delivery of insight to decision-makers, who consume the output of continuous analysis to ensure that they have the information they need to make proactive decisions as business requirements and opportunities change.
Proactive intelligence encompasses multiple categories of products. Specifically, we see automated decision intelligence products and intelligent applications as the two delivery models for the output of continuous analytics (the ML-driven processing of operational data to automatically detect trends, anomalies and root causes).Intelligent applications refer to the new breed of intelligent enterprise (e.g., ERP, CRM, sales and HR) applications that have embedded analytics functionality and are automated by artificial intelligence and machine learning. These applications can drive business process improvements by reducing friction and accelerating time to insight and action by finance, marketing, logistics and human resources decision-makers.
The use of intelligent applications with embedded analytics and AI/ML functionality will be critical to driving business decision-making going forward, fostering new organizational and management dynamics, and driving business process improvements in business functions such as finance, marketing, logistics and human resources.
451 Research's Voice of the Enterprise: Workforce Productivity & Collaboration, Tech Evaluations survey found that 35% of all respondents plan to adopt AI/ML for intelligent business applications in the next 24 months, with planned adoption highest in the financial services, software and IT services, and retail sectors.
Automated decision intelligence products, meanwhile, rely on proactive intelligence to detect anomalies and automate root-cause diagnostics in order to proactively explain what changes are occurring and why. Automated decision intelligence products make use of machine learning processes to provide decision-makers with information about what has happened – as well as root-cause analysis to identify why it has happened – and recommend how they should respond, automatically providing machine-learning-driven recommendations to facilitate strategic decision-making.
This information can be presented to decision-makers in multiple formats, including automated visualizations, natural language descriptions and recommended actions. Additionally, businesses can leverage machine learning to measure the speed of decision-making and track the real-life impact of recommended actions, with a view to closing the feedback loop in order to further improve anomaly detection, forecasting and recommendations based on identified business impact.
It is worth noting that techniques such as automated visualizations, natural language descriptions and recommended actions are also being used by purveyors of business intelligence products aimed at data analysts. One of the key differences with proactive intelligence is that it is used to provide information directly to decision-makers – via phone, email or application alerts – that does not require them to break their usual workflow to go searching for insight.
While automated decision intelligence products are general-purpose, intelligent applications are naturally department- and application-specific (e.g., HR, finance, supply chain, etc.). Both are examples of data, insight and recommendations being proactively delivered to business decision-makers to accelerate actionable insight.
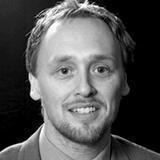
Matt Aslett is a Research Director with responsibility for the Data, AI & Analytics Channel at 451 Research, a part of S&P Global Market Intelligence. This Channel includes operational and analytic databases, Hadoop, grid/cache, stream processing, data integration, data governance and data management, as well as data science and analytics, machine learning and AI. Matt's own primary area of focus currently includes data abstraction, virtualization and analytics acceleration, data culture and data literacy, data streaming, and streaming data integration, as well as hybrid cloud data processing.
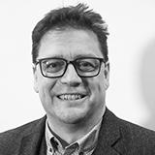
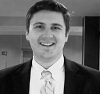