Introduction
The 451 Take
MLOps
These assets are categorically different than traditional applications, and thus, require additional considerations. For example, unlike software code, predictive models erode over time – a phenomenon known as model drift – and adoptees must therefore monitor model performance after deployment. In determining how frequently to sample and retrain deployed models, organizations must weigh many factors, such as the time of data scientists, the compute costs of model retraining and the business risks associated with a wayward prediction. This simple example, which only includes managing one model in deployment, demonstrates the exponential complexity of MLOps. Many organizations we have spoken with recently have 10 or 20 models in production now, but expect that to grow to hundreds within a year or so.
In 2020 we expect MLOps to move from an industry buzzword to a set of formalized best practices. Additionally, we suspect many providers of machine learning platforms will roll out additional features or tools targeting the management of models.
Accountable AI
The transformative potential of AI technology is awesome – it inspires both excitement and a healthy degree of fear, especially as it becomes integrated into more facets of society. One common refrain across the industry is the imperative nature of ensuring these proliferating AI systems are not harmful to the objects of their decision-making. In other words, the technology must be accountable to the humans it impacts.
AI Infrastructure
While much of the AI buzz focuses on use cases, the underlying infrastructure to build these applications is also critical. Each stage of the machine learning process – data management and preparation, model training or inferencing – places unique demands on enterprise IT systems. Additionally, the magnitude of data feeding an AI system can reach petabyte scale, and given the experimental nature of developing machine learning models, AI workloads are not always predictable. Most enterprise adoptees of AI have already begun to develop strategies around their AI infrastructure. They know that the success or failure of this critical technology depends, at least in part, on having adequate (or even superior) infrastructure.
Sustainable AI
As more enterprises adopt the technology, AI workloads are increasing in both size and frequency, resulting in more compute resources being used for AI. As a research study from OpenAI demonstrates, this trend is particularly acute in the area of cutting-edge research, where after decades of alignment with Moore's Law (which predicted a doubling in processing power every two years), the compute used for landmark AI experiments is doubling every few months. One of the main reasons for this change is deep learning. Another driver of power consumption will be the automation of key stages of machine learning development, which makes it easier to consume vast numbers of processor cycles with the click of a button. However, some vendors claim their automated ML is in fact extremely efficient; a lot depends on the chip architecture being used, such as CPU, FPGAs, GPU or TPU.
New, Innovative Use Cases
It may seem obvious to say there will be new use cases for AI in 2020, since we see new use cases emerging every day as it is. But we expect to see organizations using machine learning to solve increasingly complex use cases as they move from applying AI to problems to which they have previously applied deterministic software approaches (i.e., rules-based ones) to new use cases that were not previously solvable using software alone. We saw evidence of this in our 2019 Voice of the Enterprise AI & Machine Learning Use Cases survey, where 37% of financial services companies said they intend to use AI to solve compliance problems in the future, compared with 28% today. Other prominent examples include drug discovery, the perennial problem of enterprise search and even the design of chips for AI workloads.
Market Maturation
There will always be a need for general-purpose AI platforms – a set of tools that can be used to build and deploy any sort of AI application. But this isn't something that can be attempted by every AI vendor, although many try. We expect a dose of realism to take hold among the AI startup community – the vendors themselves, as well as their investors – as they realize that there will not be a sustainable market for hundreds of general-purpose AI platforms, and there may only be room for a handful eventually. The filleting of the public cloud market is an example of this, and the three leading providers there – AWS, Google and Microsoft – all offer general-purpose AI platforms.
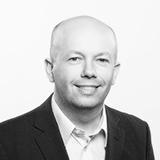
Nick Patience is 451 Research’s lead analyst for AI and machine learning, an area he has been researching since 2001. He is part of the company’s Data, AI & Analytics research channel but also works across the entire research team to uncover and understand use cases for machine learning. Nick is also a member of 451 Research’s Center of Excellence for Quantum Technologies.
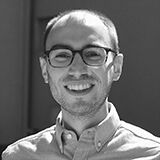
Jeremy Korn is an Associate Analyst at 451 Research. He graduated from Brown University with a BA in Biology and East Asian Studies and received
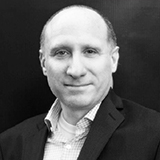
Keith Dawson is a principal analyst in 451 Research's Customer Experience & Commerce practice, primarily covering marketing technology. Keith has been covering the intersection of communications and enterprise software for 25 years, mainly looking at how to influence and optimize the customer experience.