Published: May 7, 2020
Introduction
We track use cases for AI and machine learning across multiple industries as part of our Voice of the Enterprise AI & Machine Learning survey-based research. Our most recent survey was performed in November 2019, right on the cusp of the COVID-19 outbreak. So, while we didn't ask respondents how COVID-19 would affect their use of AI, we can make informed projections about the leading and lagging use cases. In this report, we look at three industries: manufacturing, energy and media/entertainment. An additional report looks at use cases in healthcare, financial services and retail.
The 451 Take
Manufacturing

Energy
The energy industry has suffered the double blow of COVID-19 causing huge slumps in demand, coupled with the collapse in oil prices. According to the International Energy Agency, demand could drop across the world by 6% in 2020, which would be seven times the rate after the 2008 Great Recession. Its forecast issued on April 30 predicted oil demand would fall by 9.3 million barrels per day and pointed out that power demand declined by about 20% in countries with full lockdowns in place.
Picking up on that last point, predicting energy consumption was the second-most-popular machine learning use case in our survey, with 38% of respondents choosing it and 55% doing so for two years' hence. We expect interest to accelerate driven by the experience during the pandemic, where we have seen surges in home usage offset by slumps in office, shop and factory energy consumption. Usage peaks have shifted; the peak in Europe now comes later in the morning instead of early evening or early morning. In the US, peak is still in the evening as the lockdown is less uniformly enforced and has even eased in some states. AI technology can help energy providers adapt to new consumption trends or even help operators predict how the relaxation of social distancing measures will impact energy consumption going forward.
Smart grid distribution was another popular use case with 34% of respondents choosing it now and 52% in the future. When demand is reduced, demand response is key to keeping the grid operating at a stable frequency – e.g., 60Hz in the US and parts of Asia, 50Hz in most of the rest of the world. With normal grid distribution patterns no longer providing much value, AI can help grid distribution managers manage the new normal.
Risk analysis is something utilities are well prepared for – arguably better than some governments. They have business continuity plans for pandemics and have acted upon them. Some 34% of respondents see risk analysis as a machine learning use case now, rising to 52% in two years' time. We expect when we ask that question again, those numbers will be higher, considering that companies could want to turn to AI to help them evaluate and manage the proliferating risks created by these uncertain times.
Media and Entertainment
The outlook could be grim for many segments of the media industry, including some parts that have seen a massive boost as the pandemic took hold. For example, take OTT services such as Netflix and Hulu. A survey of 974 US adults at the end of March by Kagan, a media research group within S&P Market Intelligence, found that if respondents lost their job and had to reduce expenses, the media service they would be most likely to cancel was streaming service subscriptions at 37%. Traditional TV services, i.e., cable or satellite, came in second at 28% with home internet and finally mobile phone being the last two things to be cut, at 21% and 14%, respectively. Given that 52% in the same survey said they were 'very' or 'somewhat concerned' about losing their job (and 6% already had lost theirs), the picture for media in the short term at least is a dicey one, despite the surge in subscriptions to OTT services during the pandemic and lockdown.
Given all that, where does machine learning fit in? The use cases we identified in the 451 survey in Q419 in the media and entertainment industry are pertinent to the issues facing the sector both during and after the COVID-19 pandemic. The second-most-popular use case (32%) was network optimization, which has been crucial for OTT services such as Netflix that have had to downgrade their video quality to avoid crashing networks in certain countries, something they have achieved seamlessly from the customers' point of view. This is also due in part to the increasingly software-driven networks these companies employ, which themselves use machine learning.
At the time of the survey, respondents cited Intelligent archive search and asset identification as having the joint best prospects, leaping from 27% choosing it as a current use case to 51% in two years' time. For sports broadcasters having to rely on showing famous sporting events from the past during a pandemic where no sports are being played, this has been the source of their content.
The other use case to have strong prospects (a 24-percentage-point increase from current to future use case) was automated content creation. Given how much cost cutting will be happening at media companies of various types, using machine-learning-driven natural-language generation may be one of the key ways media companies stay in business. This use case may also be buoyed by the need of content providers to generate alternative forms of content or accelerate post-production of captured content to keep up with customer demand for new entertainment.
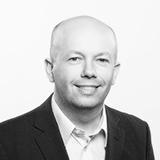
Nick Patience is 451 Research’s lead analyst for AI and machine learning, an area he has been researching since 2001. He is part of the company’s Data, AI & Analytics research channel but also works across the entire research team to uncover and understand use cases for machine learning.
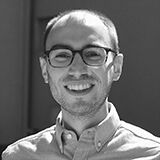
Jeremy Korn is an Associate Analyst for the Data, AI & Analytics Channel at 451 Research, where he covers artificial intelligence and machine learning in the enterprise. In particular, he focuses on the legal and ethical challenges raised by these emerging technologies. In addition, Jeremy helps lead the Voice of the Enterprise: AI and Machine Learning survey, which provides qualitative insights into AI adoption, use cases and infrastructure.
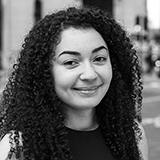
Rachel Dunning is a Research Associate at 451 Research. Prior to joining 451 Research, she graduated from Fitchburg State University Magna Cum Laude with a BS in Cognitive Psychology and Economics. While attending school, she gained exposure to research methodology and data analytics through her involvement in several academic research projects. She is conversationally fluent in German.